Predictive Modeling of Pesticides Reproductive Toxicity in Earthworms Using Interpretable Machine-Learning Techniques on Imbalanced Data
Uko Maran
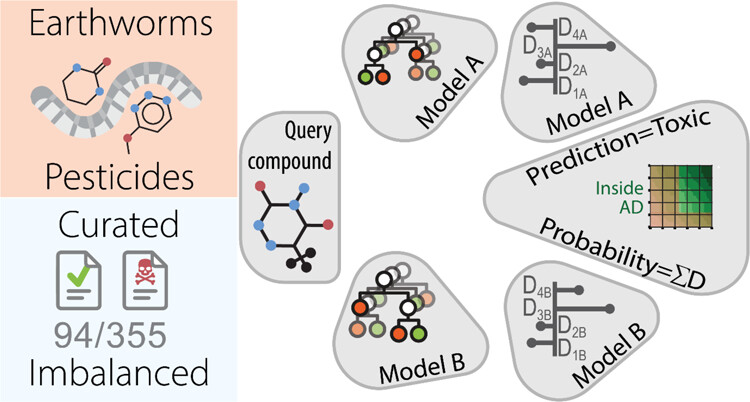
Earthworms play an important role in maintaining soil fertility and in the formation and maintenance of water and aeration systems. This makes the earthworm a key indicator organism in soil ecosystems. This in turn makes the reproductive toxicity of chemical compounds (e.g. pesticides) to earthworms a desired property of determination and makes computational models necessary for descriptive and predictive purposes. Thus, the aim was to develop an advanced Quantitative Structure–Activity Relationship modeling approach for this complex property with imbalanced data. The approach integrated gradient-boosted decision trees as classifiers with a genetic algorithm for feature selection and Bayesian optimization for hyperparameter tuning. An additional goal was to analyze and interpret, using SHAP values, the structural features encoded by the molecular descriptors that contribute to pesticide toxicity and nontoxicity, the most notable of which are solvation entropy and a number of hydrolyzable bonds. The final model was constructed as a stacked ensemble of models and combined the strengths of the individual models. Evaluation of this model with an external test set of 147 compounds demonstrated a well-defined applicability domain and sufficient predictive capabilities with a Balanced Accuracy of 77%. The model representation follows FAIR principles and is available on QsarDB.org.
Article: https://doi.org/10.1021/acsomega.4c09719
FAIR data and models: http://dx.doi.org/10.15152/QDB.263